Dramatic success in machine learning has led to a surge of Artificial Intelligence (AI) applications. Continued advances promise to produce autonomous systems that will perceive, learn, decide, and act on their own. However, the effectiveness of these systems is limited by the machine’s current inability to explain their decisions and actions to human users. We’re facing challenges that demand more intelligent, autonomous and symbiotic systems. Explainable AI—especially explainable machine learning—will be essential if future warfighters are to understand, appropriately trust, and effectively manage an emerging generation of artificially intelligent machine partners.
What is explainable AI?
Explainable artificial intelligence, or XAI, is a set of processes and methods that allow us to comprehend and trust the results and output created by machine learning algorithms. Explainable AI is used to describe an AI model, its expected impact, and potential biases. It helps characterize model accuracy, fairness, transparency, and outcomes in AI-powered decision making. Explainable AI is crucial for an organization in building trust and confidence when putting AI models into production. AI explainability also helps an organization adopt a responsible approach to AI development.
As AI becomes more advanced, humans are challenged to comprehend and retrace how the algorithm came to a result. The whole calculation process is turned into what is commonly referred to as a “black box" that is impossible to interpret. These black-box models are created directly from the data. And, not even the engineers or data scientists who create the algorithm can understand or explain what exactly is happening inside them or how the AI algorithm arrived at a specific result.
There are many advantages to understanding how an AI-enabled system has led to a specific output. Explainability can help developers ensure that the system is working as expected, it might be necessary to meet regulatory standards, or it might be important in allowing those affected by a decision to challenge or change that outcome.
Making AI transparent
Explainable AI (XAI) is an emerging field in machine learning that aims to address how black box decisions of AI systems are made. This area inspects and tries to understand the steps and models involved in making decisions. XAI is thus expected by most of the owners, operators and users to answer some hot questions like: Why did the AI system make a specific prediction or decision? Why didn’t the AI system do something else? When did the AI system succeed and when did it fail? When do AI systems give enough confidence in the decision that you can trust it, and how can the AI system correct errors that arise?
One way to gain explainability in AI systems is to use machine learning algorithms that are inherently explainable. For example, simpler forms of machine learning such as decision trees, Bayesian classifiers, and other algorithms that have certain amounts of traceability and transparency in their decision making can provide the visibility needed for critical AI systems without sacrificing too much performance or accuracy. More complicated, but also potentially more powerful algorithms such as neural networks, ensemble methods including random forests, and other similar algorithms sacrifice transparency and explainability for power, performance, and accuracy.
However, there is no need to throw out the deep learning baby with the explainability bathwater. Noticing the need to provide explainability for deep learning and other more complex algorithmic approaches, the US Defense Advanced Research Project Agency (DARPA) is pursuing efforts to produce explainable AI solutions through a number of funded research initiatives. DARPA describes AI explainability in three parts which include: prediction accuracy which means models will explain how conclusions are reached to improve future decision making, decision understanding and trust from human users and operators, as well as inspection and traceability of actions undertaken by the AI systems. Traceability will enable humans to get into AI decision loops and have the ability to stop or control their tasks whenever the need arises. An AI system is not only expected to perform a certain task or impose decisions but also has a model with the ability to give a transparent report of why it took specific conclusions.
Why do we need Explainable AI?
Design interpretable and inclusive AI
Build interpretable and inclusive AI systems from the ground up with tools designed to help detect and resolve bias, drift, and other gaps in data and models. AI Explanations in AutoML Tables, AI Platform Predictions, and AI Platform Notebooks provide data scientists with the insight needed to improve datasets or model architecture and debug model performance. The What-If Tool lets you investigate model behavior at a glance.
Deploy AI with confidence
Grow end-user trust and improve transparency with human-interpretable explanations of machine learning models. When deploying a model on AutoML Tables or AI Platform, you get a prediction and a score in real-time indicating how much a factor affected the final result. While explanations don’t reveal any fundamental relationships in your data sample or population, they do reflect the patterns the model found in the data.
Performance and scalability
Simplify your organization’s ability to manage and improve machine learning models with streamlined performance monitoring and training. Easily monitor the predictions your models make on AI Platform. The continuous evaluation feature lets you compare model predictions with ground truth labels to gain continual feedback and optimize model performance.
What are the benefits of explainable AI?
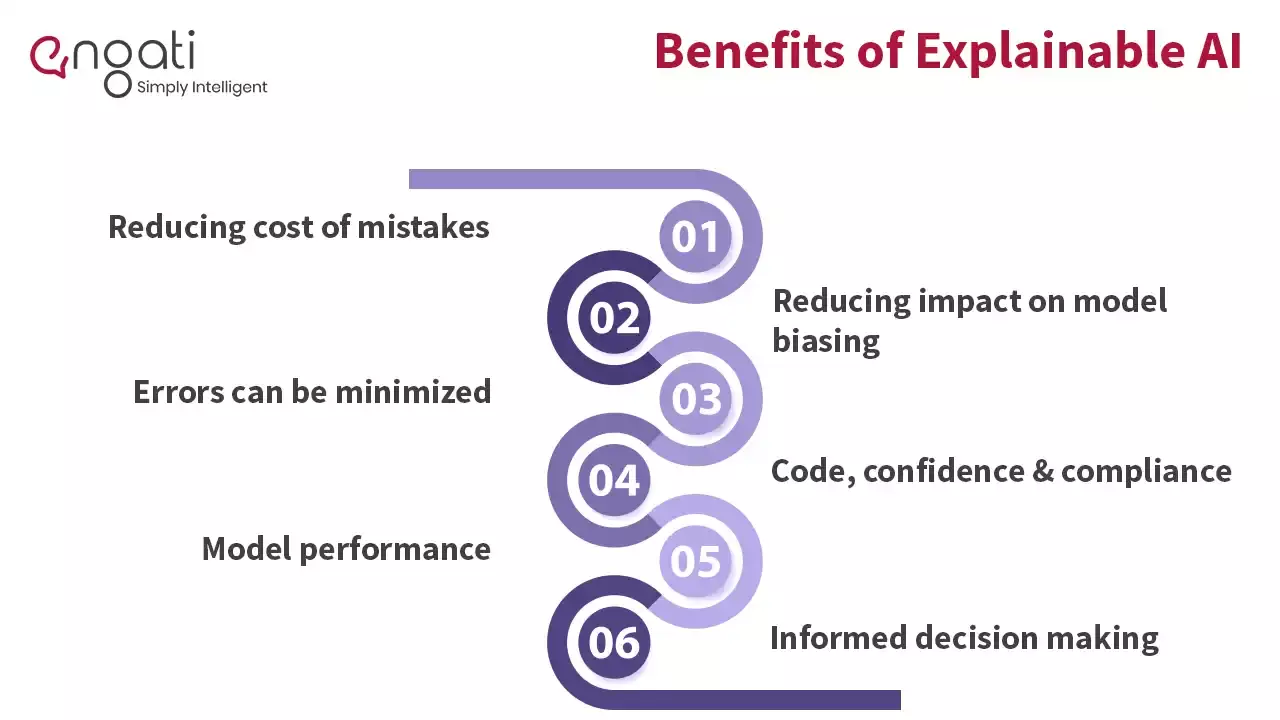
There are significant business benefits of building interpretability into AI systems. As well as helping address pressures such as regulation, and adopt good practices around accountability and ethics, there are significant benefits to be gained from being on the front foot and investing in explainability today.
The greater the confidence in the AI, the faster and more widely it can be deployed. Your business will also be a stronger position to foster innovation and move ahead of your competitors in developing and adopting new generation capabilities.
Decision-sensitive fields such as Medicine, Finance, Legal, etc., are highly affected in the event of wrong predictions. Oversight over the results reduces the impact of erroneous results & identifying the root cause leading to improving the underlying model. As a result things such as AI writers become more realistic to use and trust over time.
AI models have shown significant evidence of bias. Examples include gender Bias for Apple Cards, Racial Bias by Autonomous Vehicles, Gender, and Racial bias by Amazon Rekognition. An explainable system can reduce the impact of such biased predictions cause by explaining decision-making criteria.
AI models always have some extent of error with their predictions, and enabling a person who can be responsible and accountable for those errors can make the overall system more efficient
Every inference, along with its explanation, tends to increase the system's confidence. Some user-critical systems, such as Autonomous vehicles, Medical Diagnosis, the Finance sector, etc., demands high code confidence from the user for more optimal utilization.
For compliance, increasing pressure from the regulatory bodies means that companies have to adapt and implement XAI to comply with the authorities quickly.
One of the keys to maximising performance is understanding the potential weaknesses. The better the understanding of what the models are doing and why they sometimes fail, the easier it is to improve them. Explainability is a powerful tool for detecting flaws in the model and biases in the data which builds trust for all users. It can help verifying predictions, for improving models, and for gaining new insights into the problem at hand. Detecting biases in the model or the dataset is easier when you understand what the model is doing and why it arrives at its predictions.
The primary use of machine learning applications in business is automated decision making. However, often we want to use models primarily for analytical insights. For example, you could train a model to predict store sales across a large retail chain using data on location, opening hours, weather, time of year, products carried, outlet size etc. The model would allow you to predict sales across my stores on any given day of the year in a variety of weather conditions. However, by building an explainable model, it’s possible to see what the main drivers of sales are and use this information to boost revenues.
Where can explainable be used?
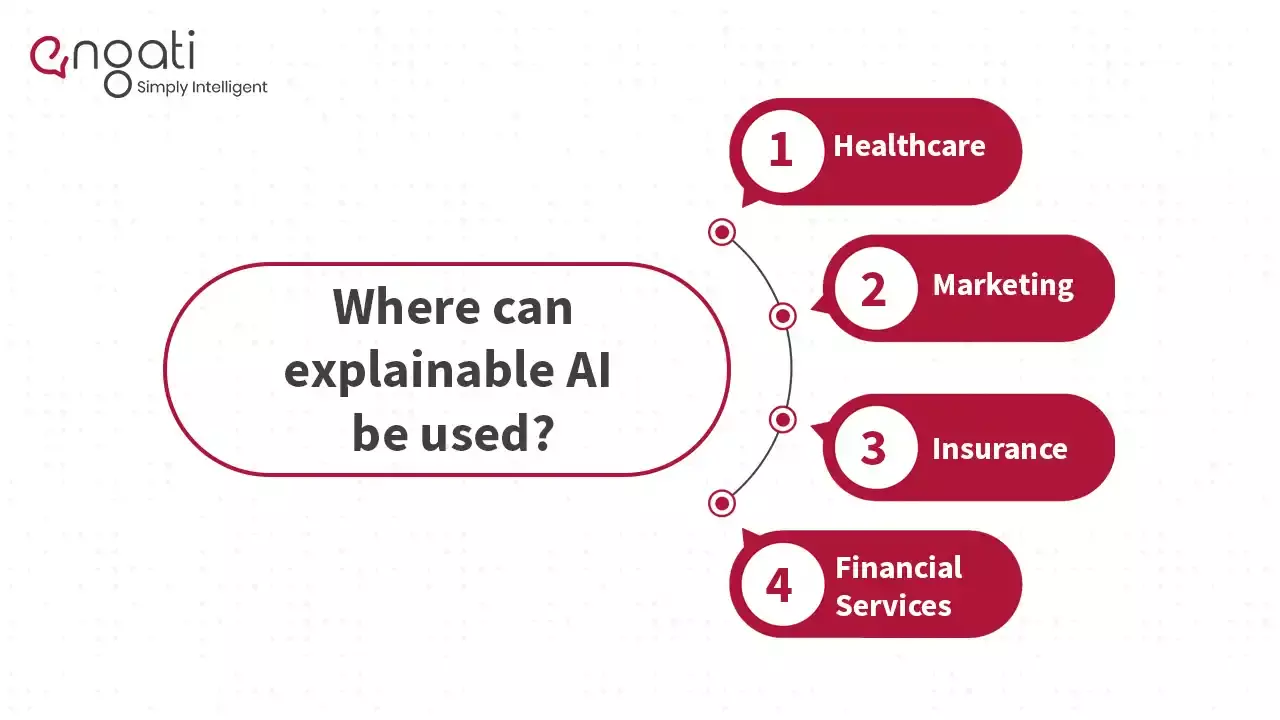
The number of industries and job functions that are benefiting from XAI are endless. So, I will list a few specific benefits for some of the main functions and industries that use XAI to optimize their AI systems.
Healthcare
Machine learning and AI technology are already used and implemented in the healthcare setting. However, doctors are unable to account for why certain decisions or predictions are being made. This imposes limitations on how and where AI technology can be applied.
With XAI, doctors are able to tell why a certain patient is at high risk for hospital admission and what treatment would be most suitable. This enables doctors to act based on better information.
Marketing
AI and machine learning continue to be an important part of companies’ marketing efforts—including the impressive opportunities to maximize marketing ROI through the business insights provided by them.
With such powerful information that helps guide marketing strategies, marketers must ask themselves “How can I trust the reasoning behind the AI’s recommendations for my marketing actions?”
With XAI, marketers are able to detect any weak spots in their AI models and mitigate them, thus getting more accurate results and insights that they can trust. This is possible as XAI provides them with a better understanding of expected marketing outcomes, the reasons behind the recommended marketing actions, and keys to improving efficiency with faster and more accurate marketing decisions and increasing their marketing ROI while decreasing potential costs.
Insurance
With the insurance industry having considerable impacts, insurers must trust, understand, and audit their AI systems to optimize their full potential.
XAI has proven to be a game-changer for many insurers. With it, insurers are seeing improved customer acquisition and quote conversion, increased productivity and efficiency, and decreased claims rates and fraudulent claims.
Financial Services
Financial institutions are actively leveraging AI technology. They look to provide their customers with financial stability, financial awareness, and financial management.
With XAI, financial services provide fair, unbiased, and explainable outcomes to their customers and service providers. It allows financial institutions to ensure compliance with different regulatory requirements while following ethical and fair standards.
Just a few ways XAI benefits the financial industry include improving market forecasting, ensuring credit scoring fairness, discovering factors associated with theft to reduce false positives, and decreasing potential costs caused by AI biases or errors.
Get ahead
Thanks for reading! We hope you found this article helpful. Ready to level-up your business? Click here!