What is data governance?
Data governance is a collection of processes, roles, policies, standards, and metrics that ensure the effective and efficient use of information in enabling an organization to achieve its goals. It establishes the processes and responsibilities that ensure the quality and security of the data used across a business or organization. Data governance defines who can take what action, upon what data, in what situations, using what methods.
A well-crafted data governance strategy is fundamental for any organization that works with big data, and will explain how your business benefits from consistent, common processes and responsibilities. Business drivers highlight what data needs to be carefully controlled in your data governance strategy and the benefits expected from this effort. This strategy will be the basis of your data governance framework.
For example, if a business driver for your data governance strategy is to ensure the privacy of healthcare-related data, patient data will need to be managed securely as it flows through your business. Retention requirements (e.g. history of who changed what information and when) will be defined to ensure compliance with relevant government requirements, such as the GDPR.

What are data governance processes?
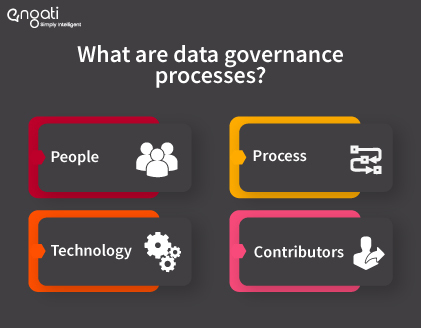
It is important to consider the people, processes, and technologies that must go into a holistic approach to data governance.
People
The first step in implementing a data governance program is to build a team and define who in your organization will be responsible for the data assets. These data owners will be accountable for the quality of the data and the support of data quality activities and initiatives company-wide.
The data team must ensure that the data governance initiatives are aligned with business needs and that the data meets their requirements. Data governance sounds like something that should be aligned with the IT portion of your organization, but in reality, it should be closely aligned with the business to ensure stakeholders can easily access the information they need to make data-driven business decisions. Without this critical communication, your organization will end up with a big data strategy as opposed to a data governance strategy.
Process
Next, data processes must be developed. These include definitions of how data will be stored, moved, changed, accessed, and secured. Control, audit, and monitoring processes must also be put in place, especially for compliance reasons in highly regulated industries.
Focusing your data governance processes on the business and its needs is very important, and the processes must reflect that.
Technology
Data governance cannot be completed with technology alone, but organizations should leverage solutions that will help with their governance initiatives. Examples include technology that will help enforce business rules, monitoring and reporting software and data quality solutions.
Contributors
Business and IT subject matter experts who provide necessary context, including business leaders, process owners, and stewards who run the upstream and downstream processes impacted by your initiative, as well as IT architects, analysts, and systems experts
What is the data governance framework?
A data governance framework creates a single set of rules and processes for collecting, storing, and using data. By doing so, the framework makes it easier to streamline and scale core governance processes, enabling you to maintain compliance, democratize data, and support collaboration—no matter how rapidly your data volumes grow.
With a data governance framework, you can ensure that your policies, rules, and definitions apply to all your data across your entire organization. You can deliver trusted data to a broad range of individuals in a variety of different roles, from business leaders to data stewards and developers. You can introduce self-service tools that enable even non-technical users to find and access the data they need for governance and analytics. And you can ensure that data is appropriately governed, transformed, and reliably delivered across all applications and analytics deployments in the cloud, on-premises, or both.
A data governance framework includes the discovery of data to create a unified view across the enterprise. This includes not only the data itself, but data relationships and lineage, technical and enterprise metadata, data profiling, data certification, data classification, data engineering, and collaboration.
A data governance framework supports the execution of data governance by defining the essential process components of a data governance program, including implementing process changes to improve and manage data quality, managing data issues, identifying data owners, building a data catalog, creating reference data, and master data, protecting data privacy, enforcing and monitoring data policies, driving data literacy, and provisioning and delivering data.
The business then uses the data governance framework to measure and monitor the results to optimize for trust, privacy, and protection. It tracks processes, data quality, and data proliferation; monitors data privacy and risk exposure; alerts you about anomalies; creates an audit trail, and facilitates issue management and workflow.
What are the different types of data governance models?
1. De-centralized Execution – Single Business Unit
This data governance model is characterized by individual business users maintaining their own master data. This model ensures that the data is created by the local users who are typically the consumers of this master data.
While this model is simpler and can make for faster master data setup, unless managed properly, users can also see huge inconsistencies in data. The following strategies and tactics can help ensure this model works effectively:
- Clearly define data ownership and limit this to a handful of experts within the organization
- Ensure clear documentation of how each field is to be populated and the meaning of each value for each field
- If budget permits, automated tools can control the consistency of data
- Set up controls and audits to quickly fix any inconsistencies
- Limit the role of data governance organization to building processes and procedures and performing periodic data audits
2. De-Centralized Execution – Multiple Business Units
This data governance model is characterized by individual business users maintaining their own master data. In this scenario, we have multiple business units working with shared customers, materials and vendors.
While this data governance model is simpler and can make for a faster master data setup, it can also result in inconsistent data with a far-reaching impact when multiple parties are involved. There is a definite need to control this model as very common side effects like duplicate master data and inconsistent data resulting in inconsistent or meaningless reporting can become troublesome. In order for this model to work effectively, it is key to:
- Leverage automated tools that can ensure the consistency of data – independent of who creates the master data
- Limit the number of fields that are maintained and let the rest of the fields be derived based on various customized profiles
- Ensure clear documentation of how each field is to be populated and what is the meaning of each value for each field
- Set up controls and audits to quickly fix any inconsistencies
- Identify controlled fields that have an impact across departments and business units, then enforce strict controls on who maintains these and clearly define what each field means
The role of the data governance organization should not be limited to building processes and procedures and performing periodic data audits, but should also include owning the automated tools and keeping them tuned to business requirements
3. Centralized Governance – Single or Multiple Business Units
This third data governance model is characterized by single or multiple business units centralizing the maintenance of master data. In this model, one central organization owns setting up master data based on requests coming from the consumers of the master data.
This data governance model can ensure a high level of control of master data, but it is often characterized by delays in setting up master data and requires a formal and larger data governance organization. As well, in this model, there is a high probability that the master data created is consistent and the introduction of changes and process improvements is quicker because there are a limited number of users setting up master data. In order to improve the model, organizations should:
- Build automated processes to provide transparency and visibility to the process of master data maintenance
- Establish KPIs for different master data requests and ensure the size of the data governance organization scales based on the requirements
- Confirm effective communication takes place between the business and master data team to ensure that master data rules are tuned to changes in the business and products
The role of the data governance organization should not be limited to processes and procedures but should also include maintenance of master data, including process adjustments to meet business needs
4. Centralized Data Governance & Decentralized Execution
This last data governance model is characterized by a centralized governance body defining the framework of controls and individual businesses creating their individual parts of master data.
centralized data governance and decentralized execution
This data governance model can ensure agility, but at the same time organizations must ensure the proper controls are in place, where needed. There is a shared responsibility in this model between the data governance organization and the business.
To effectively leverage this model, organizations must:
Identify controlled fields that have an impact across departments and business units, and then assign ownership to centralize maintenance
- Build automated tools to avoid de-duplication at the source
- Ensure a central organization mediate between various departments and business units when there is a conflict
- Automate the request process and leverage automated tools to help local businesses consistently manage data
- Set up controls and audits to quickly fix any inconsistency.