What are outliers?
In statistics, an outlier is a data point that differs significantly from other observations. An outlier may be due to variability in the measurement or it may indicate the experimental error; the latter are sometimes excluded from the data set. In a sense, this definition leaves it up to the analyst (or a consensus process) to decide what will be considered abnormal. Before abnormal observations can be singled out, it is necessary to characterize normal observations.
However, an outlier can cause serious problems in statistical analyses. Outliers can occur by chance in any distribution, but they often indicate either measurement error or that the population has a heavy-tailed distribution. In the former case, one wishes to discard them or use statistics that are robust to outliers, while in the latter case they indicate that the distribution has high skewness and that one should be very cautious in using tools or intuitions that assume a normal distribution. A frequent cause of outliers is a mixture of two distributions, which may be two distinct sub-populations, or may indicate 'correct trial' versus 'measurement error'; this is modeled by a mixture model.
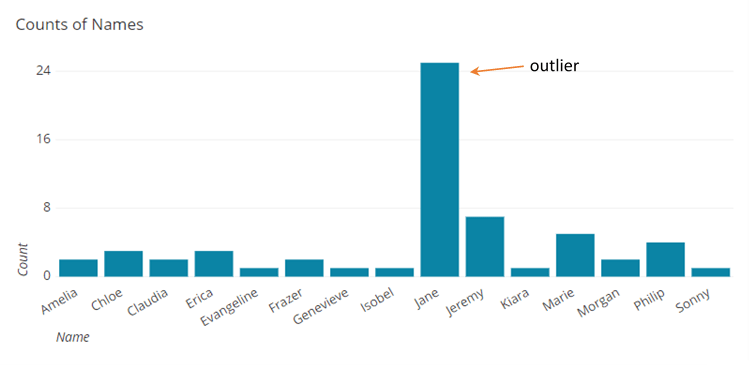
How do you determine outliers?

A careful examination of a set of data to look for outliers causes some difficulty. Although it is easy to see, possibly by use of a stemplot, that some values differ from the rest of the data, how much difference does the value have to be considered an outlier? There are a couple of methods to find what constitutes an outlier.
1. Interquartile Range
The interquartile range is what we can use to determine if an extreme value is indeed an outlier. The interquartile range is based upon part of the five-number summary of a data set, namely the first quartile and the third quartile. The calculation of the interquartile range involves a single arithmetic operation. All that we have to do to find the interquartile range is to subtract the first quartile from the third quartile. The resulting difference tells us how spread out the middle half of our data is.
2. Determining Outliers
Multiplying the interquartile range (IQR) by 1.5 will give us a way to determine whether a certain value is an outlier. If we subtract 1.5 x IQR from the first quartile, any data values that are less than this number are considered outliers. Similarly, if we add 1.5 x IQR to the third quartile, any data values that are greater than this number are considered outliers.
3. Strong Outliers
Some outliers show extreme deviation from the rest of a data set. In these cases we can take the steps from above, changing only the number that we multiply the IQR by, and define a certain type of outlier. If we subtract 3.0 x IQR from the first quartile, any point that is below this number is called a strong outlier. In the same way, the addition of 3.0 x IQR to the third quartile allows us to define strong outliers by looking at points which are greater than this number.
4. Weak Outliers
Besides strong outliers, there is another category for outliers. If a data value is an outlier, but not a strong outlier, then we say that the value is a weak outlier.
What is the difference between outliers and anomalies?
In Data Science, Anomalies are referred to as data points (usually referred to multiple points), which do not conform to an expected pattern of the other items in the data set. Anomalies are referred to as a different distribution that occurs within a distribution. Anomalies in data translate to significant (and often critical) actionable information in a wide variety of application domains.
An Outlier is a rare chance of occurrence within a given data set. In Data Science, an Outlier is an observation point that is distant from other observations. An Outlier may be due to variability in the measurement or it may indicate an experimental error.
Outliers, being the most extreme observations, may include the sample maximum or sample minimum, or both, depending on whether they are extremely high or low. However, the sample maximum and minimum are not always outliers because they may not be unusually far from other observations.
How do we remove outliers?
When considering whether to remove an outlier, you’ll need to evaluate if it appropriately reflects your target population, subject-area, research question, and research methodology. Did anything unusual happen while measuring these observations, such as power failures, abnormal experimental conditions, or anything else out of the norm? Is there anything substantially different about an observation, whether it’s a person, item, or transaction? Did measurement or data entry errors occur?
If the outlier in question is:
- A measurement error or data entry error, correct the error if possible. If you can’t fix it, remove that observation because you know it’s incorrect.
- Not a part of the population you are studying (i.e., unusual properties or conditions), you can legitimately remove the outlier.
- A natural part of the population you are studying, you should not remove it.
What do you do when you can’t legitimately remove outliers, but they violate the assumptions of your statistical analysis? You want to include them but don’t want them to distort the results. Fortunately, there are various statistical analyses up to the task. Here are several options you can try.
Nonparametric hypothesis tests are robust to outliers. For these alternatives to the more common parametric tests, outliers won’t necessarily violate their assumptions or distort their results.
In regression analysis, you can try transforming your data or using a robust regression analysis available in some statistical packages.
Finally, bootstrapping techniques use the sample data as they are and don’t make assumptions about distributions.
These types of analyses allow you to capture the full variability of your dataset without violating assumptions and skewing results.