What is statistical inference?
The practice of statistics falls broadly into two categories; descriptive or inferential. When we are just describing or exploring the observed sample data, we are doing descriptive statistics However, we are often also interested in understanding something that is unobserved in the wider population.
In these situations we have to recognise that almost always we observe only one sample or do one experiment. If we took another sample or did another experiment, then the result would almost certainly vary. This means that there is uncertainty in our result, if we took another sample or did another experiment and based our conclusion solely on the observed sample data, we may even end up drawing a different conclusion!
Statistical inference is the process of using data analysis to infer properties of an underlying distribution of probability. Inferential statistical analysis infers properties of a population, for example by testing hypotheses and deriving estimates. It is assumed that the observed data set is sampled from a larger population.
Inferential statistics can be contrasted with descriptive statistics. Descriptive statistics is solely concerned with properties of the observed data, and it does not rest on the assumption that the data come from a larger population. In machine learning, the term inference is sometimes used instead to mean "make a prediction, by evaluating an already trained model";[2] in this context inferring properties of the model is referred to as training or learning (rather than inference), and using a model for prediction is referred to as inference (instead of prediction); see also predictive inference.
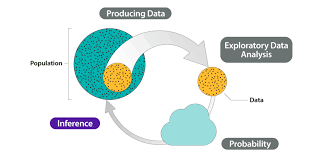
What are the 2 types of statistical inference?

There are two broad areas of statistical inference: statistical estimation and statistical hypothesis testing.
Statistical estimation
Statistical estimation is concerned with best estimating a value or range of values for a particular population parameter, and hypothesis testing is concerned with deciding whether the study data are consistent at some level of agreement with a particular population parameter. We briefly describe statistical estimation and then devote the remainder of this section to providing a conceptual overview of hypothesis testing.
There are two types of statistical estimation. The first type is point estimation, which addresses what particular value of a parameter is most consistent with the data.
The second type of statistical estimation is interval estimation. Interval estimation is concerned with quantifying the uncertainty or variability associated with the estimate. This approach supplements point estimation because it gives important information about the variability (or confidence) in the point estimate.
Statistical hypothesis testing
Hypothesis testing has a complementary perspective. The framework addresses whether a particular value (often called the null hypothesis) of the parameter is consistent with the sample data. We then address how much evidence we have to reject (or fail to reject) the null hypothesis.
What is the concept of statistical inference?
Statistical inference comprises the application of methods to analyze the sample data in order to estimate the population parameters. The basic assumption in statistical inference is that each individual within the population of interest has the same probability of being included in a specific sample. When the sample is not randomly selected. the study findings can still be generalized if the sample can be considered representative of the whole population of interest. A set of statistical methods used to infer the population parameters is performed under the assumption that the sample estimates follow a bell-shaped distribution, called normal distribution.
What is the main goal of statistical inference?
The purpose of statistical inference is to estimate this sample to sample variation or uncertainty. Understanding how much our results may differ if we did the study again, or how uncertain our findings are, allows us to take this uncertainty into account when drawing conclusions. It allows us to provide a plausible range of values for the true value of something in the population, such as the mean, or size of an effect, and it allows us to make statements about whether our study provides evidence to reject a hypothesis.
The purpose of statistical inference to estimate the uncertainty or sample to sample variation. It allows us to provide a probable range of values for the true values of something in the population. The components used for making statistical inference are:
- Sample Size
- Variability in the sample
- Size of the observed differences
What is the statistical inference procedure?
The procedure involved in inferential statistics are:
- Begin with a theory
- Create a research hypothesis
- Operationalize the variables
- Recognize the population to which the study results should apply
- Formulate a null hypothesis for this population
- Accumulate a sample from the population and continue the study
- Conduct statistical tests to see if the collected sample properties are adequately different from what would be expected under the null hypothesis to be able to reject the null cell
What are the types of statistical inference?
There are different types of statistical inferences that are extensively used for making conclusions. They are:
- One sample hypothesis testing
- Confidence Interval
- Pearson Correlation
- Bi-variate regression
- Multi-variate regression
- Chi-square statistics and contingency table
- ANOVA or T-test
Why is statistical inference important?

Inferential Statistics is important to examine the data properly. To make an accurate conclusion, proper data analysis is important to interpret the research results. It is majorly used in the future prediction for various observations in different fields. It helps us to make inference about the data. The statistical inference has a wide range of application in different fields, such as:
- Business Analysis
- Artificial Intelligence
- Financial Analysis
- Fraud Detection
- Machine Learning
- Share Market
- Pharmaceutical Sector