What is RFM analysis?
RFM analysis is a data-driven customer behavior segmentation technique that quantitatively ranks and groups customers based on their recency, frequency, and monetary value. Where RFM stands for recency, frequency, and monetary value. The basic idea of RFM analysis is to segment customers based on their purchase history, frequency of their purchases, and their overall spending in a specific time frame.
RFM analysis helps marketers to target a specific group of customers through communication, based on their behavior, creating higher response rates, increased loyalty, and customer lifetime value. RFM analysis is a helpful tool to give navigation to the marketing efforts and keep it focused on the right set of audiences.
RFM analysis categorizes each customer on the following factors:
Recency:
Recency refers to the customer's last purchase. How recent was the customer's last purchase and predicting their next purchase? Because customers who lately made a purchase will still have the product in mind and are more likely to use or buy the product again. Recency is been measured in days, weeks, or hours based on the product.
Frequency:
Frequency refers to how often customers purchase in a specific period.
Generally, if a customer buys a product, there's a possibility of more purchases followed by the first one. Marketers can increase the frequency with which customers engage and do business with the brand with the help of appropriate communication and advertisement.
Monetary:
This aspect talks about the total spending by the customer in a given period. Brands try to gauge the customers' lifetime value based on their past spending. Because customers who often spend a lot of money are more likely to spend money in the future as well. Based on the right segmentation strategy and promotional mix, brands can leverage the sale through these customers.
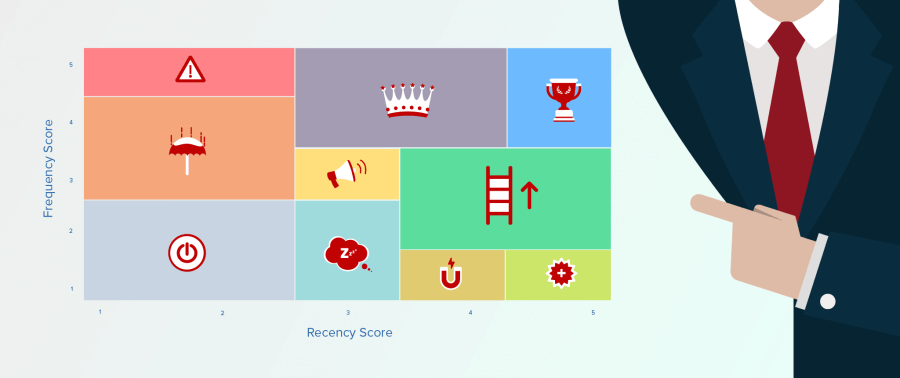
What is RFM segmentation?
RFM segmentation lets marketers narrow down their marketing efforts to the right set of audiences based on their behavior, thereby generating higher rates of responses, increased loyalty, and customer lifetime value.
This segmentation method helps brands identify the right group of customers based on past records and data.
Brands leverage the data of their existing customers like purchase history, browsing history, prior campaign response patterns, and demographics to mold their marketing strategies and channelize required communication. RFM segmentation benefits brands to save time, money, and effort while rolling out their marketing and advertisement plan, and increasing ROI on the same.
Following is the brief segmentation of customers based on the RFM segmentation analysis.
1. Champions/ best customers
These customers buy regularly, spend a lot, and are recent buyers. Brands should reward these customers and provide them with exclusive offers and discounts. They are the early adopters who buy and try new products without putting much effort into them.
2. Potential Loyalists
They are your recent customers with average frequency and have been associated with the brand for quite some time. Offer them membership or loyalty programs, upsell them and help them become your loyalists thus enhancing customer lifetime value.
3. New Customers
As the name suggests they are your customers who have a high overall RFM score but are not frequent shoppers. Start building relationships and engagement with these customers by providing onboarding support and special offers to increase their visits or frequency of purchases.
4. At-Risk Customers
These customers have been frequent and spend often on the brand, however, have not made any transactions recently. Brands can send them personalized offers and product suggestions based on their buying history.
What are the benefits of RFM analysis?

Conducting an RFM analysis on your customer base and sending personalized campaigns to high-value targets has massive benefits. RFM analysis allows you to target customers with communication that best matches their connection with your brand.
Following are the benefits of RFM analysis:
Personalization:
We are living in an era of technological advancement by which the landscape of business is changing day after another. RFM segmentation helps in hyper-personalizing the marketing communication and promotions for a different set of customers, making it more unique and personal.
Improve conversion rates:
RFM can also boost conversion rates with focused communication and increased customer engagement. Once you know the audience, it's easier to craft strategies or offers different schemes to different customers, increasing sales and frequency of purchases.
Retention:
With the help of RFM analysis brands can focus on the right set of customers at the right time or before they make a move to some other brand. Personalization helps in retaining those customers and increasing the customer lifetime value as well.
How does RFM analysis work?
RFM analysis scores customers on each of the three main dimensions (R, F, and M). Under which a score from 1 to 5 is given to each dimension, with 5 being the highest.
Thought the scoring method differs as per the implementation of the RFM analysis from organization to organization.
The grouping of three values for each client is called an RFM cell. In a simple system, organizations average these values together, then sort customers from highest to lowest to find the most valuable customers.
Highest score here denotes the most valuable customers and lower score refers to customers, brands needs to work around and turn them into value based customers.

What are the limitations of RFM analysis?
While RFM segmentation is effective, it does have certain limitations.
- If performed manually, it’s prone to human error.
- Customer demographics such as age, sex, and ethnicity are not included in RFM analysis.
- RFM analysis is also established on just a few behavioral qualities, lacking the power of advanced predictive analytics.
- Businesses may lose out on the opportunity to achieve optimum response rates on certain campaigns due to the bombardon of messages to high-ranking customers.
- Predictive techniques may identify future customer behavior that RFM analysis cannot.
- On the other hand, it can cause marketers to overlook customers with low rankings even though many of them may be worth nurturing.