What are smart responses?
A Smart Response is a tool used by Engati to streamline the current chatbot setup experience and to make it easier for you to set up a chatbot as quickly and effectively as possible.
You can set up answers to probable queries by setting up FAQs, defining Entities, uploading questions and responses in a Tabular Input format, or uploading relevant documents in Document Lookup.
Engati NLP engine has been set up and has the intelligence to search across the four types and match the most relevant response according to the query. You also have the control to change most of the settings and search preferences according to their requirements.
%20(1).jpg)
What are the key capabilities of smart responses?
The Smart Responses tool generates reply suggestions based on the full context of the conversation, not just a single message. This means the suggestions are more helpful to your users.
The tool generates replies quickly and doesn't require you to send users' messages to a remote server.
How to set up smart responses?
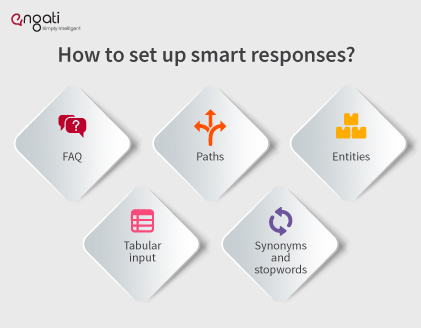
With Engati, there are 4 ways to set up smart responses.
1. FAQ
Frequently Asked Questions (FAQs) are standard query response combinations that help train your bot to handle customer queries. Engati allows you to add any number of variations in each FAQ set, thus helping make the training as broad as possible. For instance, if you have a bot for a school, it would need to be trained to answer questions like the location of the school, the timings, and the fee. These questions with all their variations and responses for each query or intent can be uploaded as FAQs. Engati takes care of the difficult task of using that to train the bot in handling these queries so that a common person may ask. The FAQs are what primarily drive your chatbot’s automated responses and our powered by our proprietary NLP engine.
There are two ways for you to add FAQs to your bot:
- One FAQ at a time: This is relevant for a scenario where the number of FAQs is limited or individual entries are made from time to time.
- Multiple FAQs in one go: There is an upload FAQ option where a user can upload a file containing all the FAQs.
2. Paths
Paths refer to complex conversational flows that involve far more interactive elements than the FAQ model could offer. They define your user journeys and help you outline how your want conversations to flow on your chatbot. You could model the entire conversation design or structure of your chatbot by making use of Engati’s conversation modeler. Every user journey or workflow on your chatbot can be built as an individual path and then you can link these individual paths appropriately using the ‘Trigger Path’ node to bring your entire chatbot together. This modular design can be rather useful if you want to trigger certain chatbot functionalities from various entry points (which could even include FAQs).
All the paths are made up of interlinked nodes. Every node performs a specific function. Some of these nodes are used to send simple messages, carousels, and multimedia like images, video, and audio. You even have a node to a message with quick responses or options as well as nodes to set attributes, identity, and much more.
You also get a lot of nodes for integrations. You can use the JSON API node to link to any standard REST API which supports various authentication modes and quick, easy ways to use the response values. There also are a lot of GUI driven native integrations like Salesforce, Google Sheets, Google Calendar, SMS providers and more.
3. Entities
Entities are data points or values that you can extract from a conversation/user query. This allows you to customize what kind of information you are collecting, how you want to associate it, and even add your own custom set of values if needed. Also, when a group of values leads to the same answer, you can use entities rather than creating FAQs for all the variables.
For instance, the enrollment procedure for all the courses in an institute is the same. A typical question could be, “How can I enroll for an Artificial Intelligence course?” Similarly, there can be n number of queries in the same context for different courses. Here rather than creating various FAQs for each subject you can make an entity set with custom values and add all the course names in it, and it will give relevant answers each time the query is triggered.
4. Tabular input
Engati provides functionality to bot builders to add a data source that has mapped Entity sets which reduces the effort to write a query for each set of information.
For Instance, in an Institute Bot, a bot builder can create custom entity sets as Course, Fees, Start date, and difficulty level.
Now define an FAQ as Q: what are the fees of the machine learning course. Tag the course with the course entity and prices with the fees entity. Add a data source as shown in the image below
Once configured, a user can ask queries for any course for which the mapped value will be displayed from the data source.
5. Synonyms and stopwords
Text Preprocessing is the action of transforming text to make it ready for Natural Language Processing. Engati’s proprietary NLP engine, e.Sense automatically handles various aspects of text preprocessing, including stemming, spelling mistakes handling, context handling, synonyms handling, stopwords reduction, parts of speech handling, and more.
Of these, Synonyms and Stopwords often have a very domain-specific context. Hence, Engati provides a way for you to be able to manage these easily for your bot. The section below describes the configuration options available for you in more detail.